Medical Imaging Aided Disease Detection System
With the growing emphasis on preventive medicine, the mortality rates of various types of
cancer have shown a consistent decline due to early detection through screening. However, lung cancer poses a unique
challenge as it often remains asymptomatic in its early stages, silently progressing until the tumor reaches a size
that compresses the airway, resulting in symptoms such as wheezing, coughing, or distant metastasis. By the time these
symptoms manifest, the disease has typically advanced to stage III or IV. National statistics indicate that the
five-year survival rate for lung cancer in the first stage is approximately 90%. However, if diagnosis is delayed
until the fourth stage, the five-year survival rate plummets to a mere 10%. Since 2017, lung cancer has surpassed
colorectal cancer as the leading cause of cancer-related deaths. Therefore, early diagnosis and treatment are pivotal
in improving both the cure rate and overall survival rate for lung cancer patients.
Low-dose computed tomography (LDCT) has emerged as the most effective tool for evidence-based early lung cancer screening and diagnosis. The government and healthcare institutions have long been committed to promoting nationwide screening programs for lung cancer. In line with this, as of July 2022, the Ministry of Health and Welfare's Health Promotion Administration has initiated public subsidies for LDCT screening targeting high-risk populations susceptible to lung cancer.
Nonetheless, interpreting LDCT scans poses a substantial challenge for physicians. Amidst the analysis of hundreds of high-resolution images featuring intricate vascular networks, accurately identifying minute pulmonary nodules measuring in millimeters and manually assessing their size and characteristics becomes an arduous task. The process involves managing vast volumes of image data, requiring a significant allocation of human resources, ensuring long-term quality control, and incurring substantial costs. Consequently, relying solely on traditional manual image interpretation raises concerns about the trade-off between the labor-intensive nature of the task and the public health imperative.
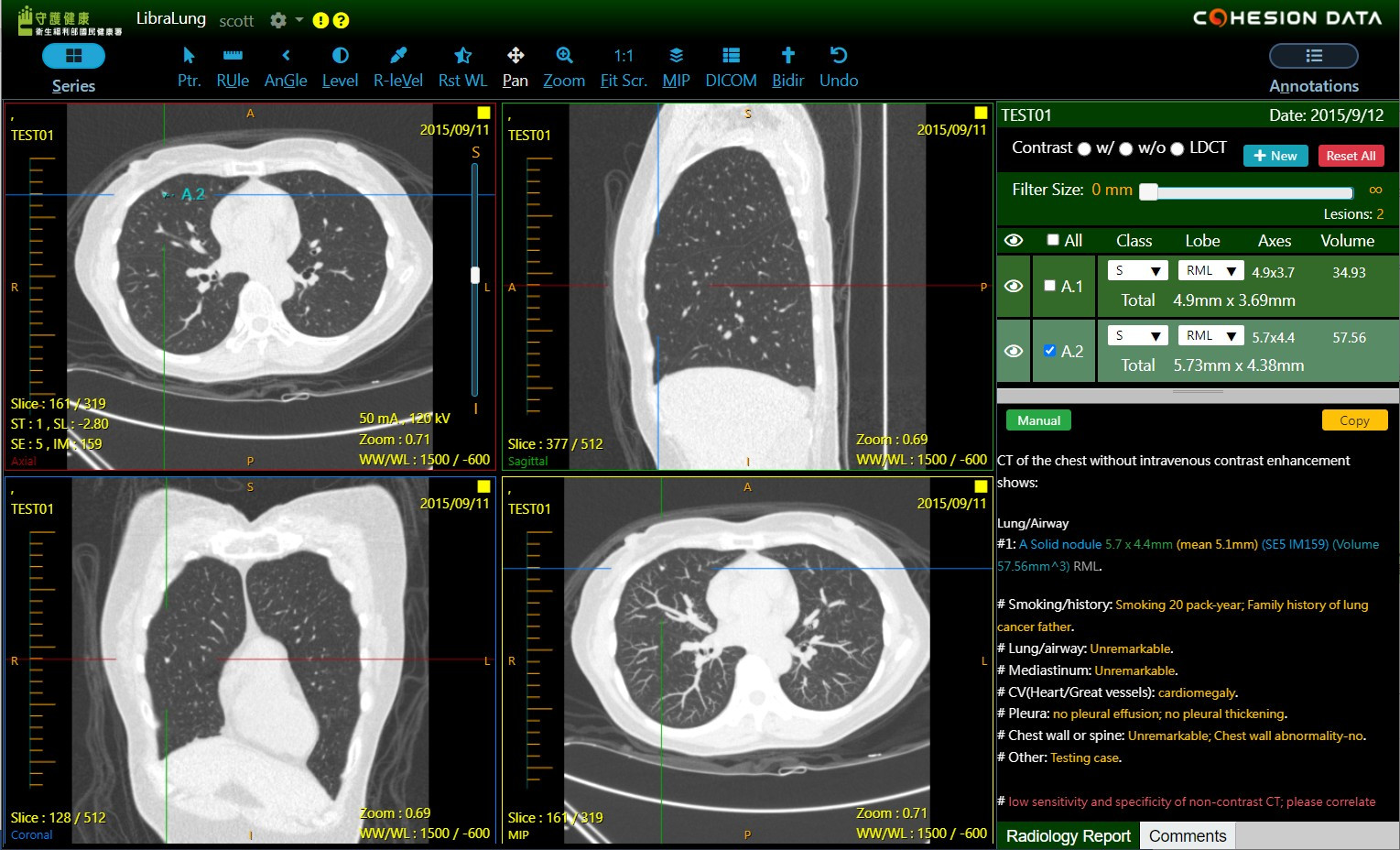

Reduce errors in physician-written reports
Report with one click
Convenience for follow up
The Health Promotion
Administration version of "LibraLung" is provided free of charge to medical institutions in
Taiwan. Medical institutions are welcome to email the service team libralung@cohesiondata.com for more
information.
The software is currently undergoing review by Taiwan's Food and Drug Administration, and we hope to accelerate its application and development in the medical field, and to work together with the medical community to improve the survival rate of lung cancer and enhance the quality of lung cancer prevention and treatment for the people of Taiwan.
Low-dose computed tomography (LDCT) has emerged as the most effective tool for evidence-based early lung cancer screening and diagnosis. The government and healthcare institutions have long been committed to promoting nationwide screening programs for lung cancer. In line with this, as of July 2022, the Ministry of Health and Welfare's Health Promotion Administration has initiated public subsidies for LDCT screening targeting high-risk populations susceptible to lung cancer.
Nonetheless, interpreting LDCT scans poses a substantial challenge for physicians. Amidst the analysis of hundreds of high-resolution images featuring intricate vascular networks, accurately identifying minute pulmonary nodules measuring in millimeters and manually assessing their size and characteristics becomes an arduous task. The process involves managing vast volumes of image data, requiring a significant allocation of human resources, ensuring long-term quality control, and incurring substantial costs. Consequently, relying solely on traditional manual image interpretation raises concerns about the trade-off between the labor-intensive nature of the task and the public health imperative.
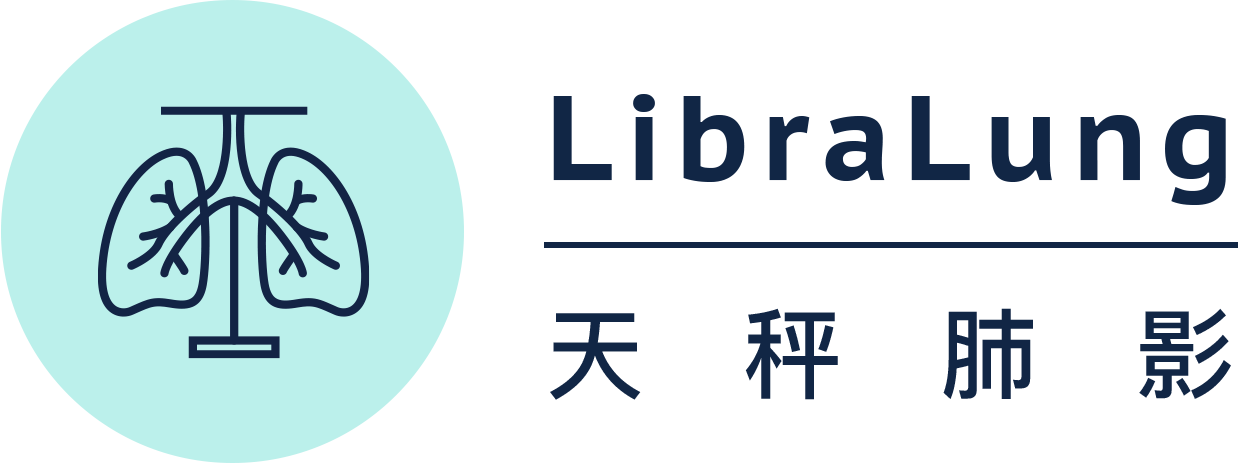
The "LibraLung" system, a computer-aided detection system for lung imaging,
utilizes artificial intelligence (AI) to assist physicians in the intricate and meticulous task of identifying and
interpreting pulmonary nodules. By alleviating the long-term workload pressure on physicians, this system aids in
various aspects of the complex reporting process, including the laborious data input and the prompt generation of
clear reports. It not only facilitates the immediate tasks at hand but also provides a powerful tool for subsequent
comparative analysis and tracking of lesions. By automating these information-intensive tasks, LibraLung replaces
tedious manual operations, thereby enhancing the efficiency of healthcare delivery and establishing an irreplaceable
high-quality operational environment.
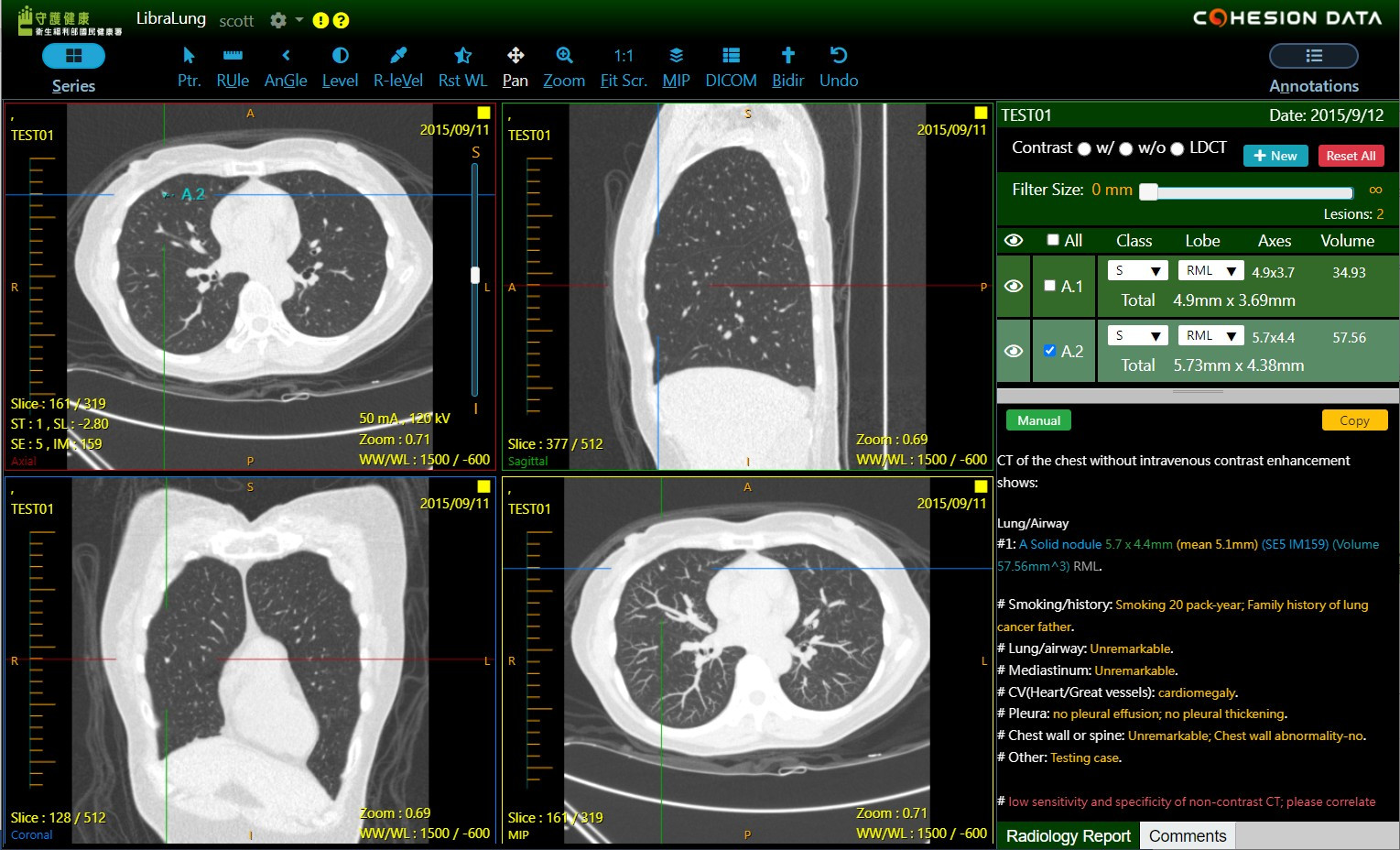
According to clinical trials conducted at medical centers

With the assistance of LibraLung, a computer-aided
diagnosis system for pulmonary nodules, the percentage of experienced physicians correctly identifying pulmonary
nodules in a sample of cases increased by 25% compared to those without the system.

With the assistance of LibraLung, a computer-aided
detection system, the accuracy of image interpretation by physicians has increased by 25% compared to
interpretation by physicians alone.

- A 31% increase in consistency in the classification of lung nodules.
- An increase of at least 33% in consistency in identifying the affected lobe of the lung.
- Improved consistency and accuracy in marking, which helps with tracking lesion localization and VDT (volume doubling time) calculations for diagnosis.

- With the assistance of LibraLung, physicians can reduce manual measurement of lung nodules and complete reports more efficiently. Clinical trials at medical centers have shown that the average reading time for CT scans decreased by 29% for young physicians, 43% for mid-career physicians, and 15% for senior physicians, resulting in an overall improvement in reading efficiency of 29%.
- The system also utilizes the commonly used PACS-Viewer interface, allowing physicians to switch between systems quickly. In a satisfaction survey conducted among specialized radiologists at three medical centers, the average satisfaction rating after using the system was above 4.5 out of 5.
AI-assisted physician diagnosis
Automatically generate information related to
pulmonary nodules, perform identification, segmentation, and classification. Thus, it’s provide the
following information:
- Which type of pulmonary nodules (solid, part-solid, non-solid)
- Which location of the pulmonary lobe (left upper lobe, left lower lobe, right upper lobe, right middle lobe, right lower lobe)
- Maximum diameter, average diameter, volume
- Image location (slice, slice thickness (ST), slice location (SL), series number (SE), instance number (IM))
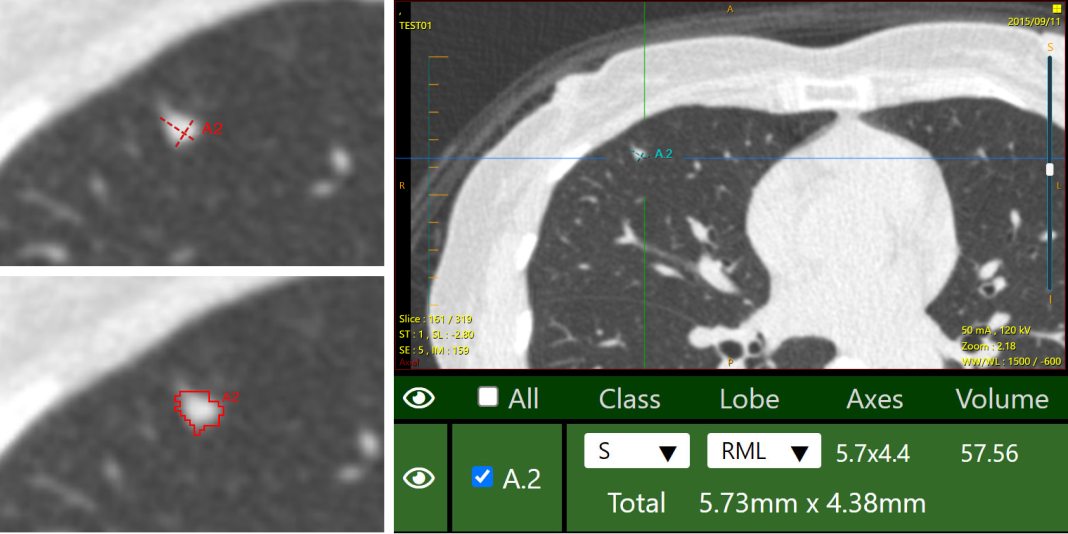



Generating auto-structured medical record

Reduce errors in physician-written reports

Report with one click

Convenience for follow up
Rigorous clinical trials. Verify the ground truth
meticulously.
The "LibraLung" system has been meticulously developed by a team of
16 radiologists specializing in diagnostic imaging, in collaboration with AI data experts. This collaborative effort has resulted in a highly sensitive model for lung nodule detection, achieving an impressive performance with a sensitivity rate of up to
92% (Avg. FP/scan=
0.6). The accuracy of pulmonary nodule type classification is as high as
0.91, and the sensitivity is as high as
0.88. For the classification of the lobe location of pulmonary nodules, the accuracy and sensitivity were even higher, reaching
0.99. The model has been trained using a combination of international clinical databases and local case studies, encompassing approximately
2000 cases and
6300 lung nodules. Each nodule has undergone a rigorous three-step validation process, incorporating the clinical expertise of at least
3 physicians.
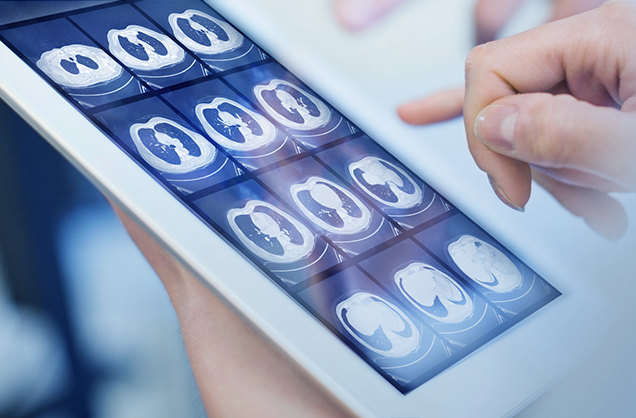
The software is currently undergoing review by Taiwan's Food and Drug Administration, and we hope to accelerate its application and development in the medical field, and to work together with the medical community to improve the survival rate of lung cancer and enhance the quality of lung cancer prevention and treatment for the people of Taiwan.